Apple Says AI Can’t Reason- But Is that true?
Apple’s new GSM-Symbolic benchmark claims AI lacks true reasoning, sparking debate in the AI community. Is it pattern matching or actual intelligence? Tests may miss AI’s real-world strengths, like problem-solving and creativity. Are we even asking the right questions?
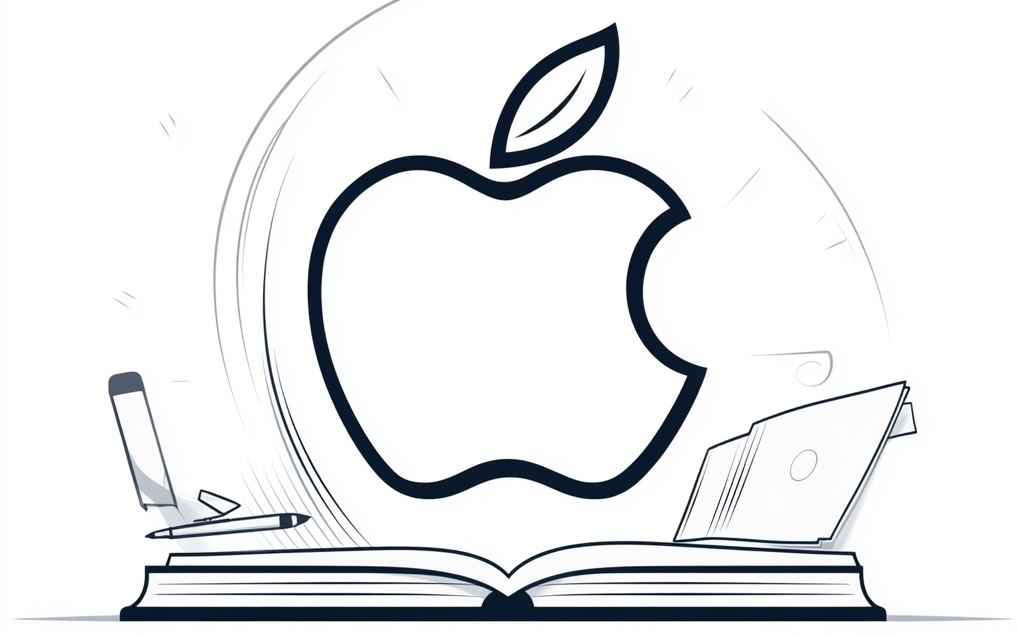
Apple’s recent GSM-Symbolic benchmark claims that advanced AI systems, like o1 Preview, fail at reasoning. The conclusion? AI can’t truly think like humans. But is this the full story? Here’s why this debate reveals more about how we misunderstand AI than about AI itself.
The Claim: AI Fails at Reasoning
Apple’s benchmark designed tricky, ambiguous tasks to test AI’s reasoning. The results showed AI falling short, leading to claims that it doesn’t truly “reason.”
But here’s the catch: AI models like ChatGPT or Claude weren’t designed to handle trick questions. They take instructions at face value, aiming to help, not to second-guess. Asking them to navigate misleading prompts is like faulting a master chef for failing a recipe that swaps salt for sugar—an unfair measure of their true abilities.
A Small Change, Big Results
AI writer Andrew Mayne put Apple’s claims to the test with a simple tweak: adding one line of instruction—“This might be a trick question. Watch for irrelevant information.”
The impact was massive: AI performance improved by 90%. This small adjustment shows how critical context is for AI. It’s not that AI lacks intelligence—it simply relies on clear, cooperative communication. This result highlights the importance of prompt engineering, the emerging art of crafting instructions to bridge human and machine thinking.
Real-World Problem-Solving
While benchmarks focus on contrived scenarios, AI’s real-world strengths tell a different story:
• Debugging Code: Acting like a detective, AI pieces together patterns and resolves issues faster than humans.
• Supporting Decisions: It draws from massive data sets, offering insights tailored to thousands of past cases.
• Enhancing Creativity: From drafting ideas to composing poetry, AI amplifies human potential in creative fields.
These aren’t “tricks.” They’re demonstrations of a new form of intelligence. Failing to measure this properly risks undervaluing what AI can already do and overestimating what it can’t.
Redefining Intelligence
Our obsession with making AI think like us limits our ability to appreciate its unique strengths. Intelligence isn’t one-size-fits-all. Just as early astronomers rethought planetary motion, we must rethink what intelligence means in the age of AI.
Instead of setting “gotcha” traps, we need tests that:
• Highlight AI’s real strengths.
• Focus on practical applications, not artificial riddles.
• Encourage collaboration between human insight and machine precision.
The real question isn’t “Can AI reason like humans?” but “Can we rethink how we evaluate intelligence itself?”
Your Takeaway
AI is changing how we solve problems, create, and collaborate. Understanding its unique capabilities—and building better ways to measure them—will shape the next chapter of human-machine interaction.
Are we asking the right questions about AI reasoning?
Link to the Paper:
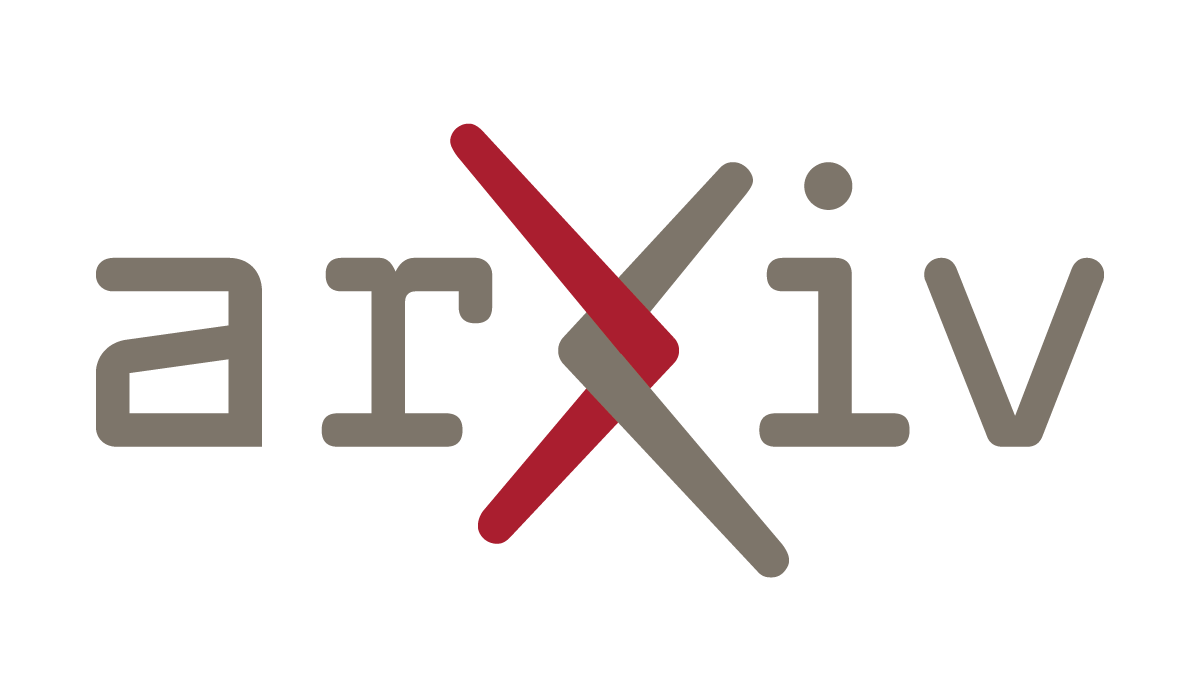